To view my full tutorial on how to use Windy for Flight Planning. See my previous blog post.
Windy has recently made a (old) new model available for Canadian aviators to use for their short term flight planning: The High-Resolution Deterministic Prediction System. For years I’ve been touting the accuracy of the European Centre for Medium-Range Weather Forecasts (ECMWF) model.
Yet the ECMWF model might have finally met its match in Canada with HRDPS. Now that it’s available in an easy to use format within Windy here is how and when you should use it. But first some background. Caution: Math ahead!
Model Resolution
The earth is huge! Covering over 510 million km2. If you were to flatten the earth into a square it would be 22.6 million km x 22.6 million km. In order to calculate weather at any given spot on the Earth we need to divide the world into sections.
This is known as the “resolution” (or grid spacing) of the model. In other words what a particular weather value will be represented in that “piece” of the earth. Let’s take a look at two models to see how resolution can affect accuracy. The GFS model which has a 22km grid spacing, and the HRDPS model with 2.5km spacing. Both are used by Environment Canada in weather forecasting.
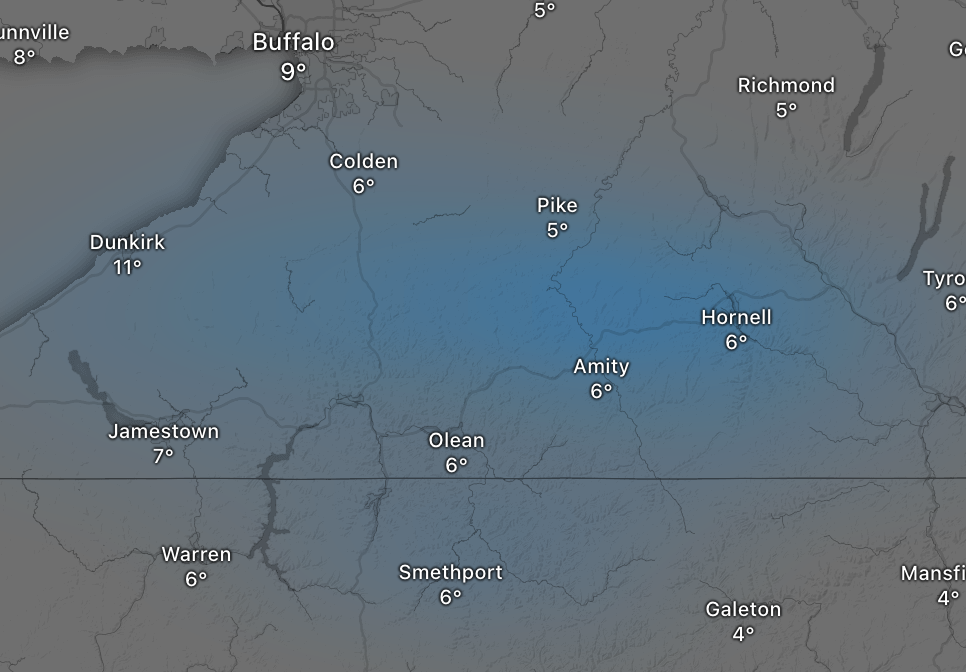
These two images are forecasting precipitation for the same region. However the higher resolution HRDPS model doesn’t show any precipitation for the town of Hornell. While the GFS model has a more “fuzzy” prediction for precipitation that does include the town of Hornell.
Resolution is important for localized phenomena, and can also help VFR pilots avoid pockets of unstable weather. But this isn’t the only thing that affects accuracy of forecast models for localized regions. The type of model that is used also plays a big role.
Spectral versus Point/Grid Models
ECMWF is a global model and therefore uses wave modes and functions to calculate variables such as temperature, winds, humidity for specific points on the earth. This is really accurate at forecasting global level events such as the location of the jet stream or large pressure systems.
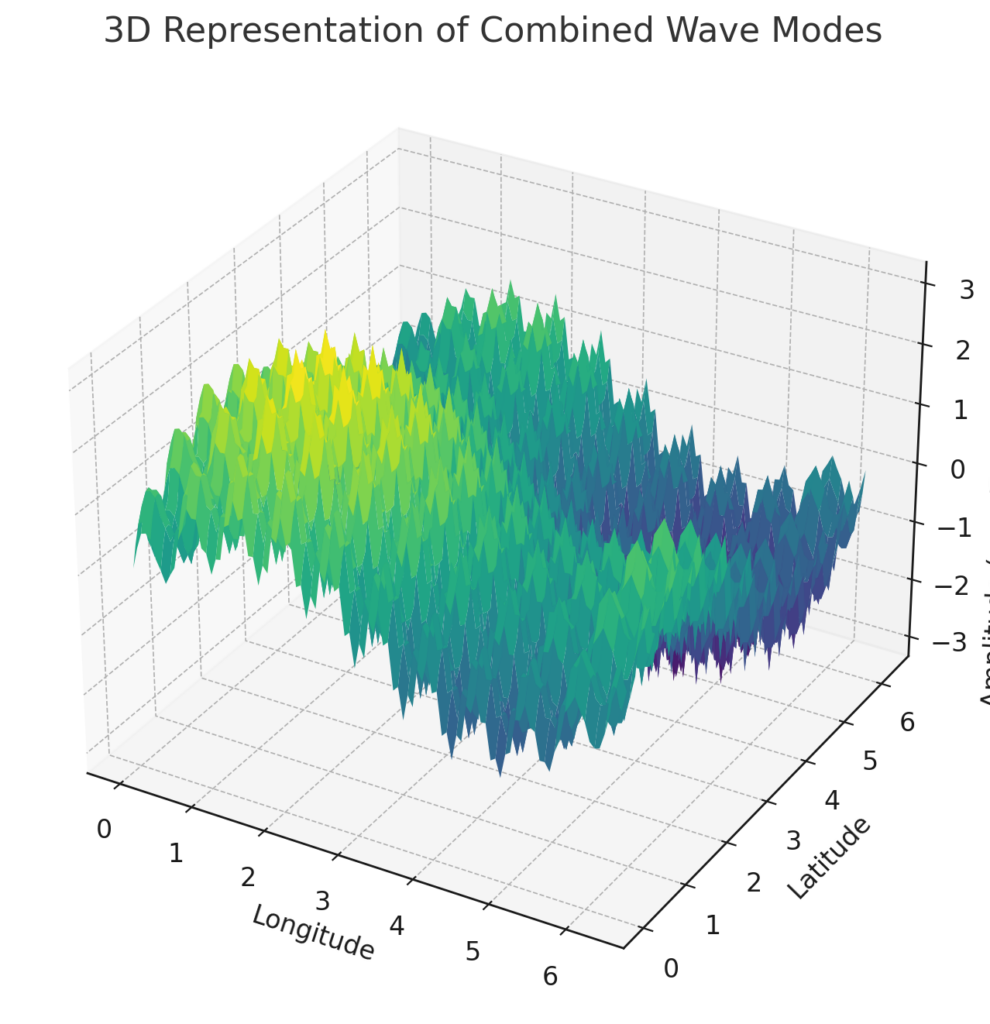
In the above example each point on this wave is a temperature at a given latitude/longitude and altitude. But this type of model has one major flaw: The closer you “zoom in” to a more localized region, the data becomes less accurate due to the nature of using waves to model your data.
Let’s consider a sharp cold front, represented at the green arrow (black line) of the two dimensional version of the same data from above:
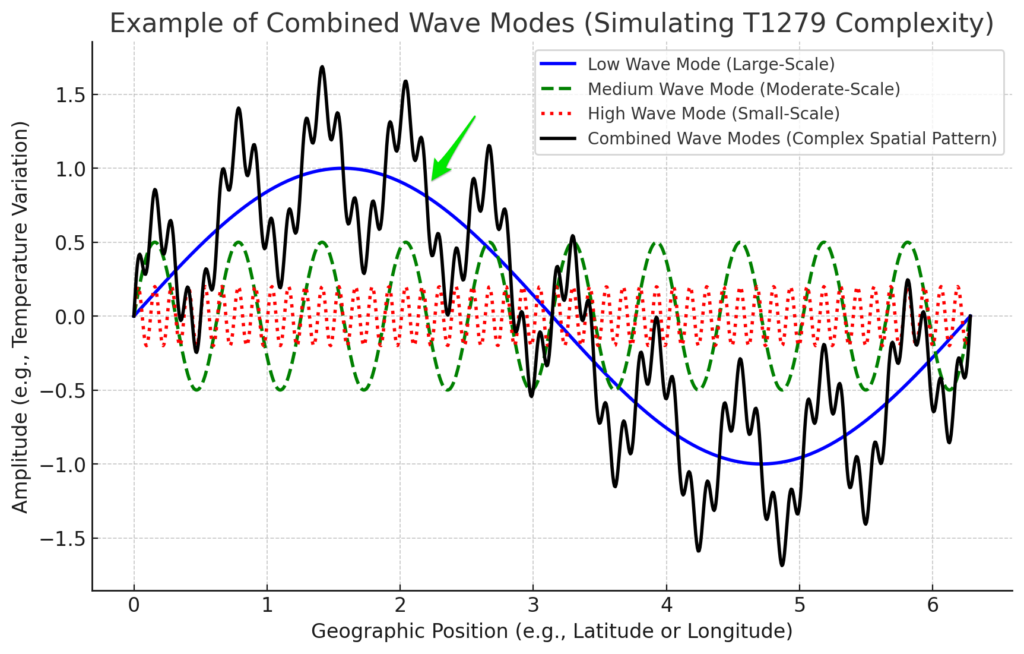
As you “zoom in” to a more localized location it’s harder to determine the slope of the line (and thus temperature gradient) of the location of the cold front. This results in the model showing a fuzzy or blurry transition between the warm and cold air masses reducing the accuracy of both the location and the intensity of the front in very localized regions.
This is where the HRDPS model comes to the rescue. Instead of using wave modes to model the weather it uses specific grid points. This provides more accurate forecasting for localized regions but at a cost: More computational power is required to forecast the weather using points instead of wave modes at high resolutions. This is why HRDPS only covers roughly 10 million km2 instead of 510 million km2 with ECMWF. Additionally, the forecast length for HRDPS is only 48 hours, where as with ECMWF its 10 days.
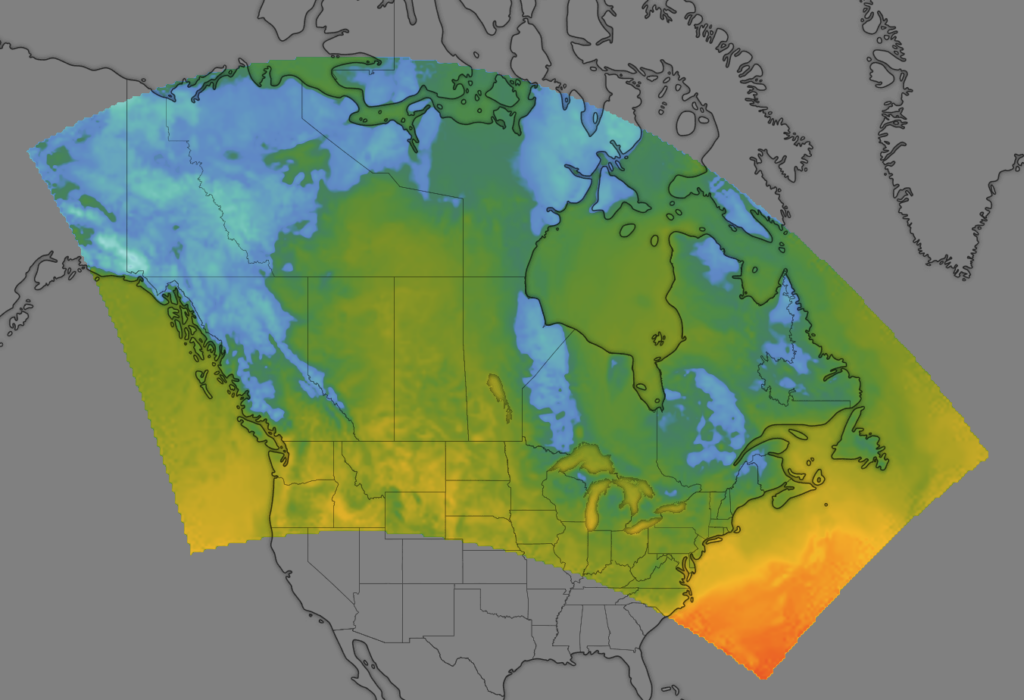
Previously, I showed you an example of how model resolution can affect how areas of precipitation are forecasted for a region. Here is an example of the difference in accuracy between a spectral model and a grid/point model. (Disregard the fact the resolution between these two models are slightly different).
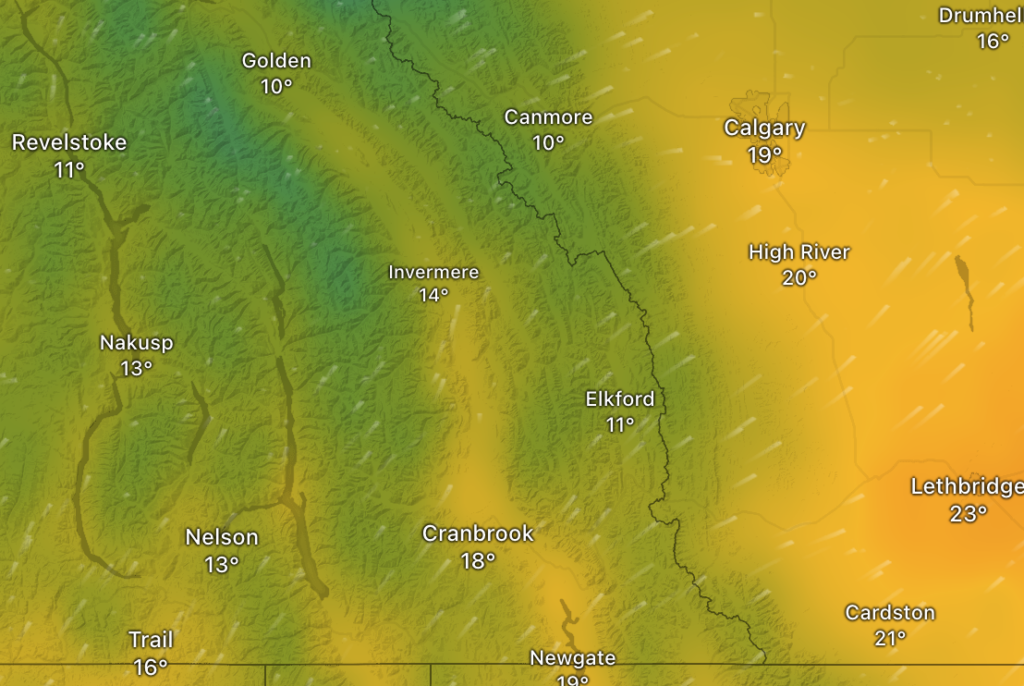
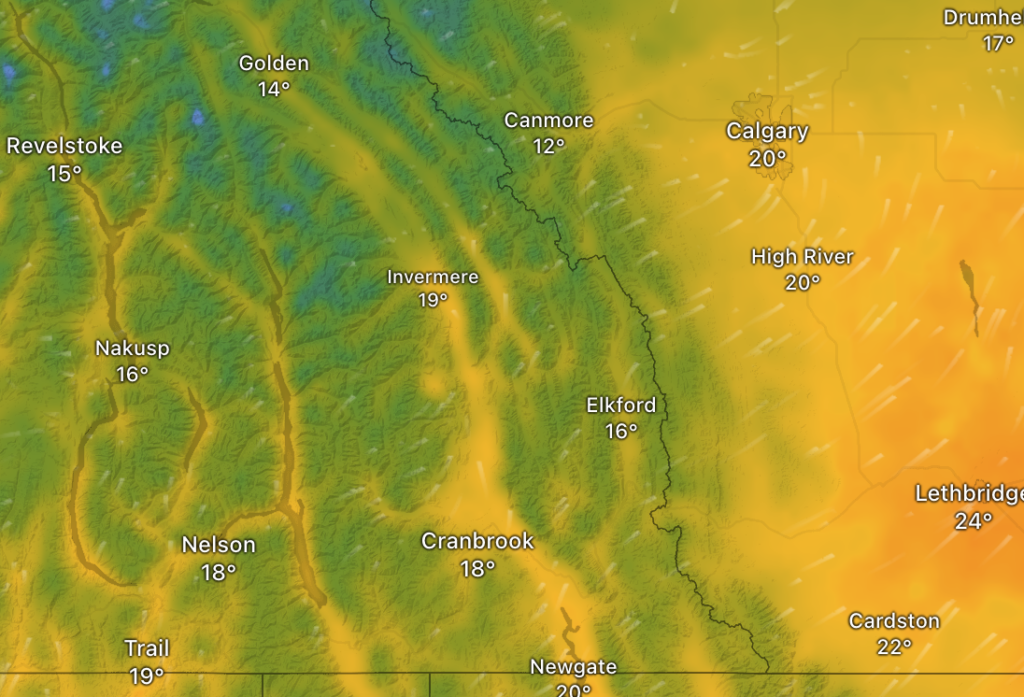
It’s clear that the HRPDS model is superior when showing steep temperature gradients. Especially when it comes to adiabatic heating on the lee side of the Rocky Mountains and in the valleys.
In other words: ECMWF excels at displaying larger global weather patterns, while HRPDS is better at displaying local phenomena.
Tag Team Time!
When it comes to accuracy level, both models have proven accuracy rates of 90% or higher 0-48 hours into the future (the farthest the HRDPS model will forecast). The ECMWF model has an accuracy rate of 80-90% with their 5 day forecast.
For you weekend warriors out there, use the ECMWF model early in the week to see what the forecast will look like for flying over the weekend. Then fine tune your planning on Thursday or Friday using the HRDPS model for a more accurate forecast for your weekend destination.
This is especially true for those of you flying in mountainous or coastal regions where HRDPS really excels at forecasting weather conditions.
One more thing…
Weather models are complex. This post only scratches the surface discussing the difference between (in my opinion) the two best models to use for flight planning. We didn’t talk about the difference between an ensemble (ECMWF) and deterministic (HRDPS) methods or how remote sensing, aircraft, buoys, and weather stations play a vital role in seeding the data for these models.
If this is something you’d be interested to know more about, let me know. Oh by the way: This was my first blog post where 100% of my research came straight from ChatGPT. For the full (hour and a half) conversation I had with the AI see this transcript.
And if you don’t have Windy premium yet. Spend the $19USD/year, it’s totally worth it. The free version only updates the models twice a day instead of ~6 times a day. Plus you get access to real time weather radar and satellite data.